What is Visual Dialog?
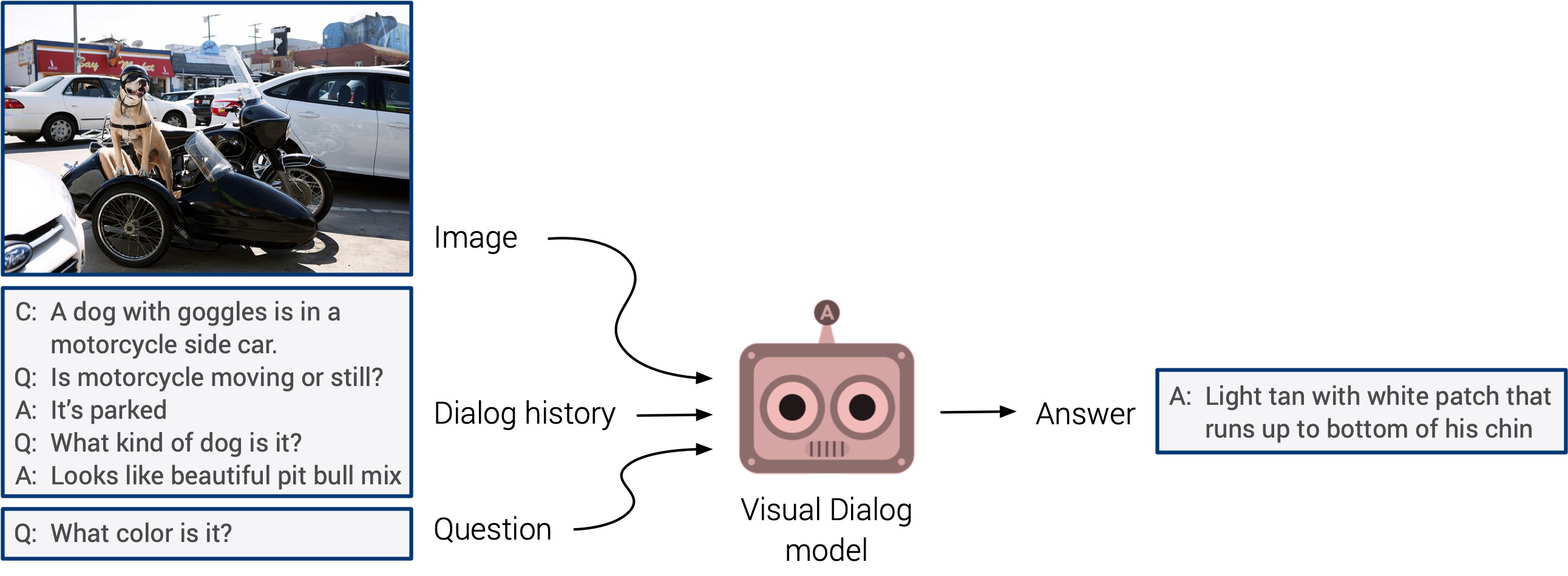
Visual Dialog requires an AI agent to hold a meaningful dialog with humans in natural, conversational language about visual content. Specifically, given an image, a dialog history, and a follow-up question about the image, the task is to answer the question.
- VisDial dataset stats:
- 120k images from COCO
- 1 dialog / image
- 10 rounds of question-answers / dialog
- Total 1.2M dialog question-answers
News
Check out this listing by @MengyuanChen21 for recent works on visual dialog![Feb 2020] Visual Dialog challenge 2020 announced on the VisDial v1.0 dataset!
[Oct 2019] PyTorch code for "Improving Generative Visual Dialog by Answering Diverse Questions" is now available!
[Jun 2019] Winners of the Visual Dialog challenge 2019 announced! Complete leaderboard here.
[Jan 2019] Visual Dialog challenge 2019 announced on the VisDial v1.0 dataset!
[Sep 2018] Winners of the Visual Dialog challenge 2018 announced! Complete leaderboard here.
[Jun 2018] Visual Dialog challenge 2018 announced on the VisDial v1.0 dataset!
[Jun 2018] PyTorch code for "Learning Cooperative Visual Dialog Agents with Deep Reinforcement Learning" is now available!
[Apr 2017] Torch code for training/evaluating Visual Dialog models, pretrained models and Visual Chatbot demo are now available!
[Mar 2017] VisDial v0.9 dataset and code for real-time chat interface used to collect data on AMT are now available!
Improving Generative Visual Dialog by Answering Diverse Questions
Visual Coreference Resolution in Visual Dialog using Neural Module Networks
Evaluating Visual Conversational Agents via Cooperative Human-AI Games
* equal contribution
Learning Cooperative Visual Dialog Agents with Deep Reinforcement Learning
* equal contribution
Visual Dialog
Acknowledgements
We thank Harsh Agrawal and Jiasen Lu for help on the AMT data collection interface; Xiao Lin, Ramprasaath Selvaraju and Latha Pemula for model discussions; Marco Baroni, Antoine Bordes, Mike Lewis, and Marc'Aurelio Ranzato for helpful discussions. Finally, we are grateful to the developers of Torch for building an excellent framework. This work was funded in part by the NSF CAREER awards to Dhruv Batra and Devi Parikh, ONR YIP awards to Dhruv Batra and Devi Parikh, ONR Grant N00014-14-1-0679 to Dhruv Batra, a Sloan Fellowship to Devi Parikh, ARO YIP awards to Dhruv Batra and Devi Parikh, an Allen Distinguished Investigator award to Devi Parikh from the Paul G. Allen Family Foundation, ICTAS Junior Faculty awards to Dhruv Batra and Devi Parikh, Google Faculty Research Awards to Dhruv Batra and Devi Parikh, Amazon Academic Research Awards to Dhruv Batra and Devi Parikh, AWS in Education Research grant to Dhruv Batra and NVIDIA GPU donations to Dhruv Batra.
License
Visual Dialog annotations and this website are licensed under a Creative Commons Attribution 4.0 International License.
Images
Visual Dialog does not own the copyright of the images. Use of the images must abide by the COCO and Flickr Terms of Use. The users of the images accept full responsibility for the use of the dataset, including but not limited to the use of any copies of copyrighted images that they may create from the dataset.
Sponsors
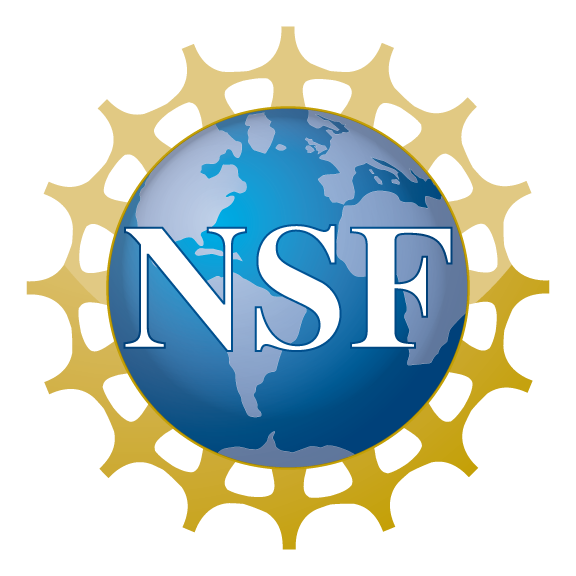
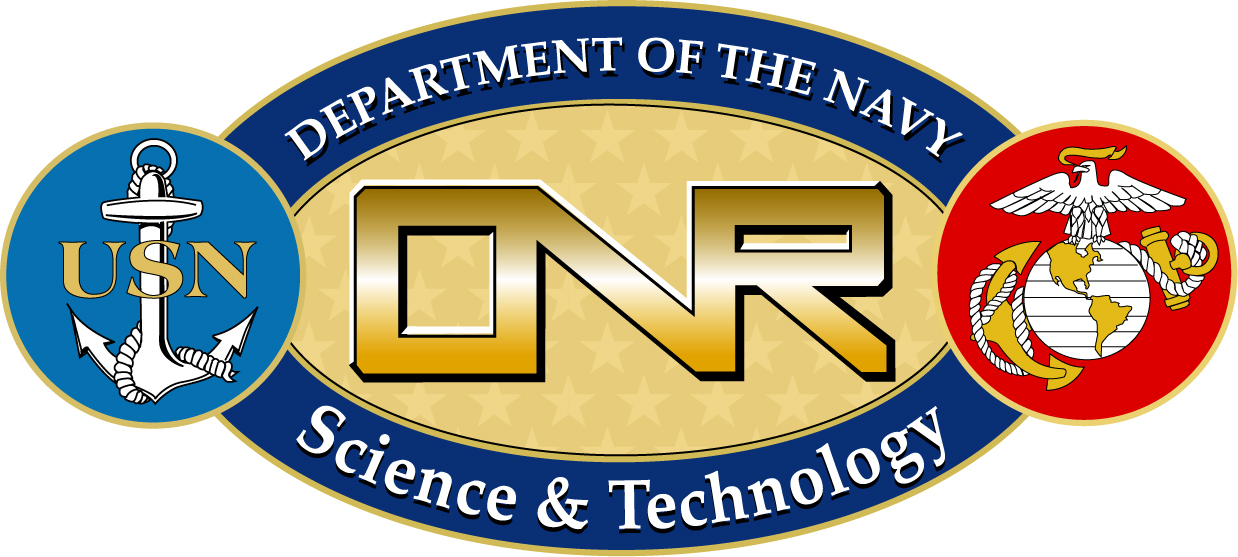
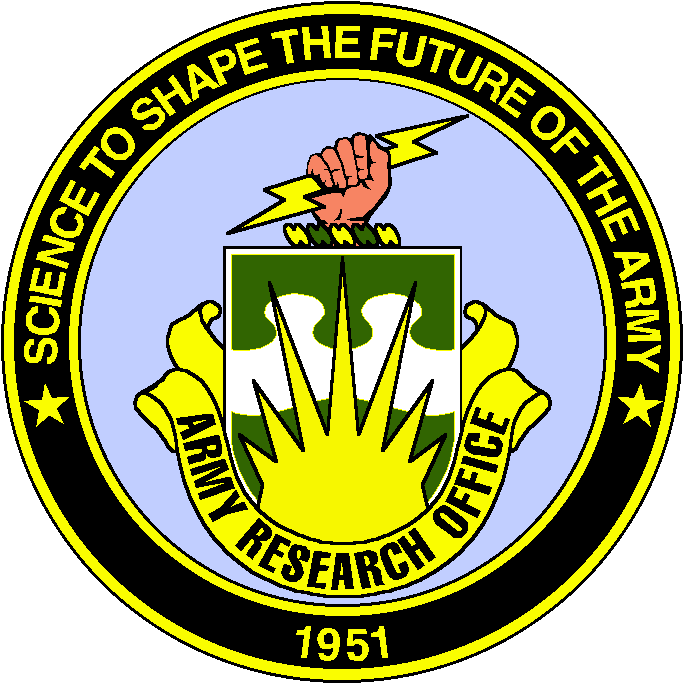
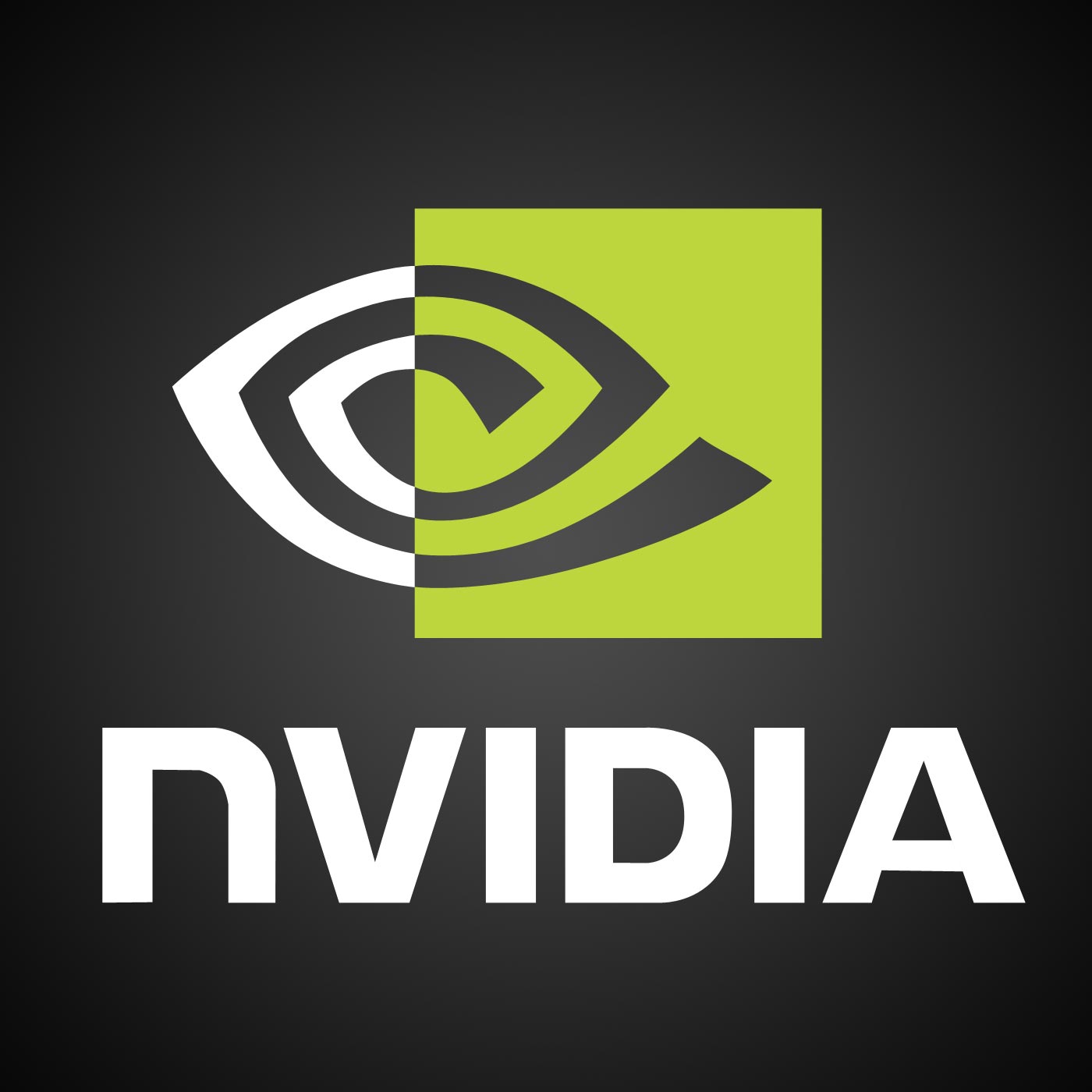

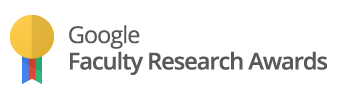
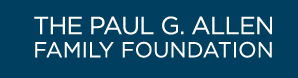
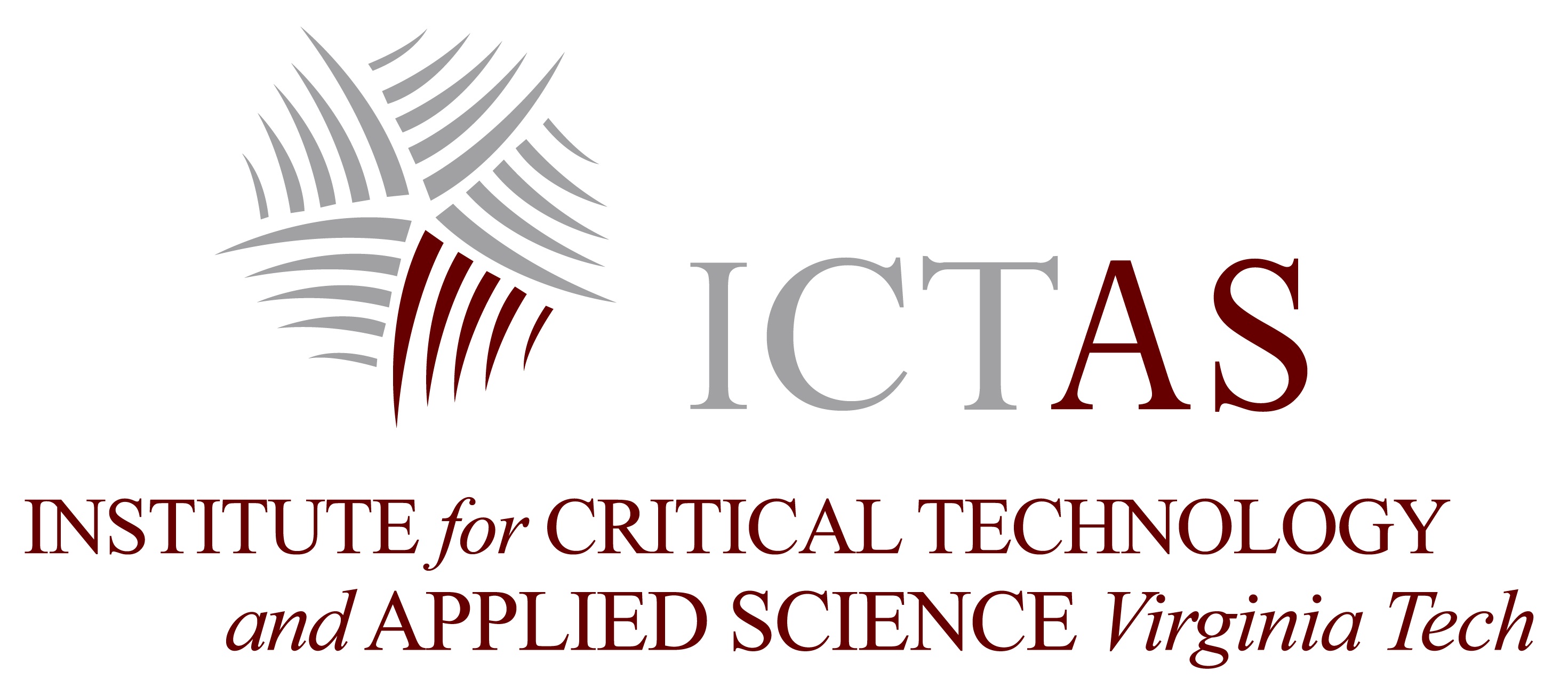